The efficient coding hypothesis states that sensory neural systems invest resources, such as energy, cells, and neural ‘wiring,’ in proportion to the expected gain of information that can be extracted from the environment. The predictions of this hypothesis can be calculated if we know the distributions of naturalistic signals that the neural system has to detect, encode, transmit and process. According to the hypothesis, our visual systems would presumably be different if they were adapted to a world containing random uncorrelated stimuli, rather than to the world populated with 3D objects that on our retinas create images full of edges, corners and texture.
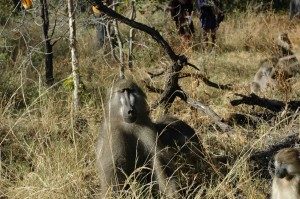
An example image from the Penn Natural Image database. We study statistical structure in natural scenes and ask if evolution has shaped our visual processing pathways to efficiently extract information from natural (but not synthetic random) stimuli.
To make quantitative predictions, we therefore study the statistics of natural scenes. In particular, we have assembled a large calibrated database of natural scenes from a riverine savannah habitat in Botswana, similar to the environment where the visual systems of our ancestors have evolved.
Existing research has dealt mostly with how low-order statistics of natural scenes, e.g. luminance distributions and pairwise correlations, influenced the design of the retina, such as its color sensitivity, the mosaic of receptive fields, and retinal cell types. In our research we want to extend the reach of efficient coding hypothesis to the domain of higher-order statistics, and to neural processing beyond sensory periphery, by asking if properties of the visual cortex are also driven by the statistics of our visual environments. We are also interested in other types of stimuli, for instance auditory and olfactory.